Defensive Distillation based Mitigations for Beamforming
Adversarial Machine Learning attacks and their mitigation methods for the next generation wireless communication systems (i.e. 5G, 6G, and 7G)
The Adversarial Security Mitigations of mmWave Beamforming Prediction Models using Defensive Distillation and Adversarial Retraining
Publication: ArXiv Preprint
Abstract. The design of a security scheme for beamforming prediction is critical for next-generation wireless networks (5G, 6G, and beyond). However, there is no consensus about protecting the beamforming prediction using deep learning algorithms in these networks. This paper presents the security vulnerabilities in deep learning for beamforming prediction using deep neural networks (DNNs) in 6G wireless networks, which treats the beamforming prediction as a multi-output regression problem. It is indicated that the initial DNN model is vulnerable against adversarial attacks, such as Fast Gradient Sign Method (FGSM), Basic Iterative Method (BIM), Projected Gradient Descent (PGD), and Momentum Iterative Method (MIM), because the initial DNN model is sensitive to the perturbations of the adversarial samples of the training data. This study also offers two mitigation methods, such as adversarial training and defensive distillation, for adversarial attacks against artificial intelligence (AI)-based models used in the millimeter-wave (mmWave) beamforming prediction. Furthermore, the proposed scheme can be used in situations where the data are corrupted due to the adversarial examples in the training data. Experimental results show that the proposed methods effectively defend the DNN models against adversarial attacks in next-generation wireless networks.
Bibtex Information
@ARTICLE{2022arXiv220208185K,
author = {Murat Kuzlu and Ferhat Ozgur Catak and Umit Cali and Evren Catak and Ozgur Guler},
title = "{The Adversarial Security Mitigations of mmWave Beamforming Prediction Models using Defensive Distillation and Adversarial Retraining}",
journal = {arXiv e-prints},
keywords = {Computer Science - Cryptography and Security, Computer Science - Artificial Intelligence, Computer Science - Machine Learning, Computer Science - Networking and Internet Architecture},
year = 2022,
month = feb,
eid = {arXiv:2202.08185},
pages = {arXiv:2202.08185},
archivePrefix = {arXiv},
eprint = {2202.08185},
primaryClass = {cs.CR},
adsurl = {https://ui.adsabs.harvard.edu/abs/2022arXiv220208185K},
adsnote = {Provided by the SAO/NASA Astrophysics Data System}
}
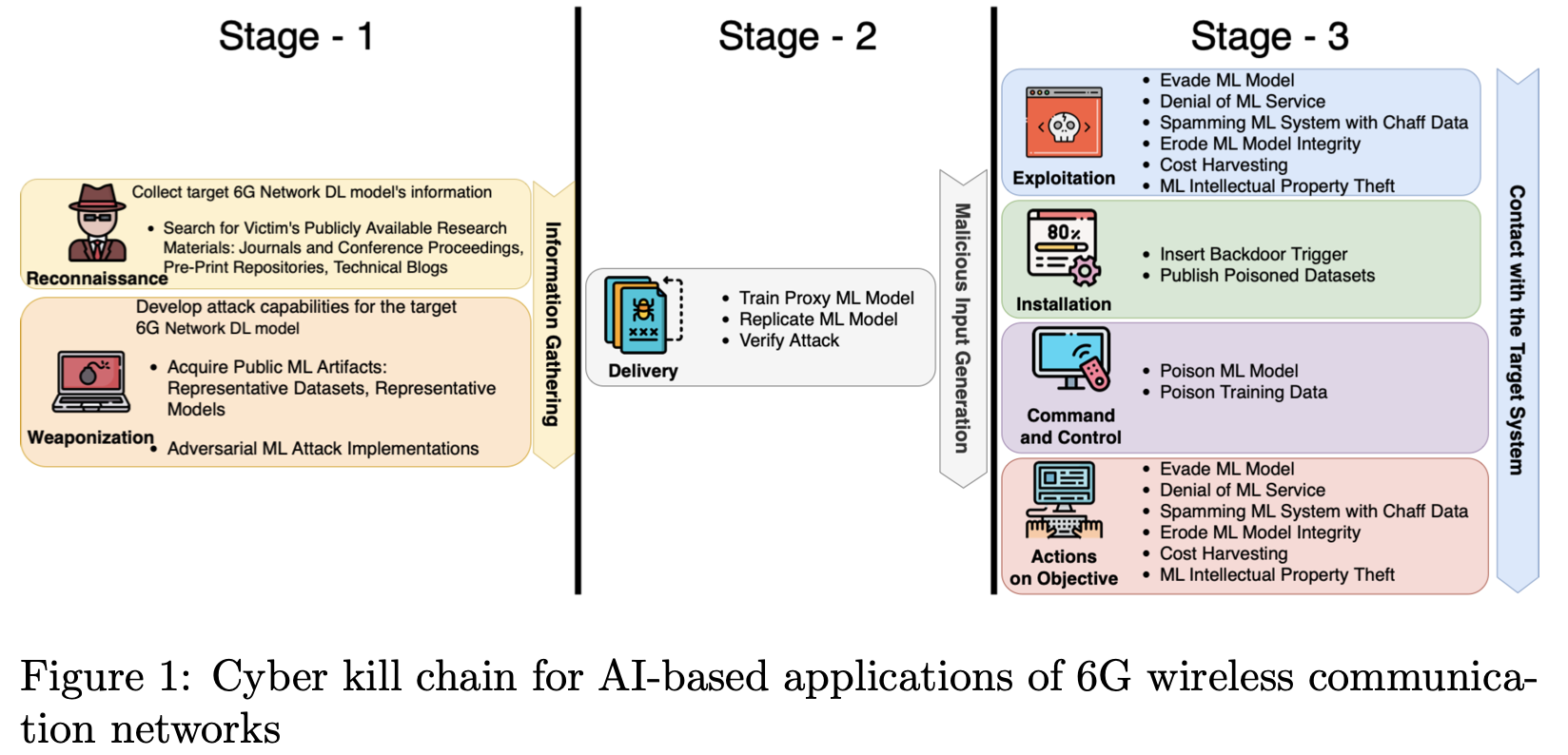
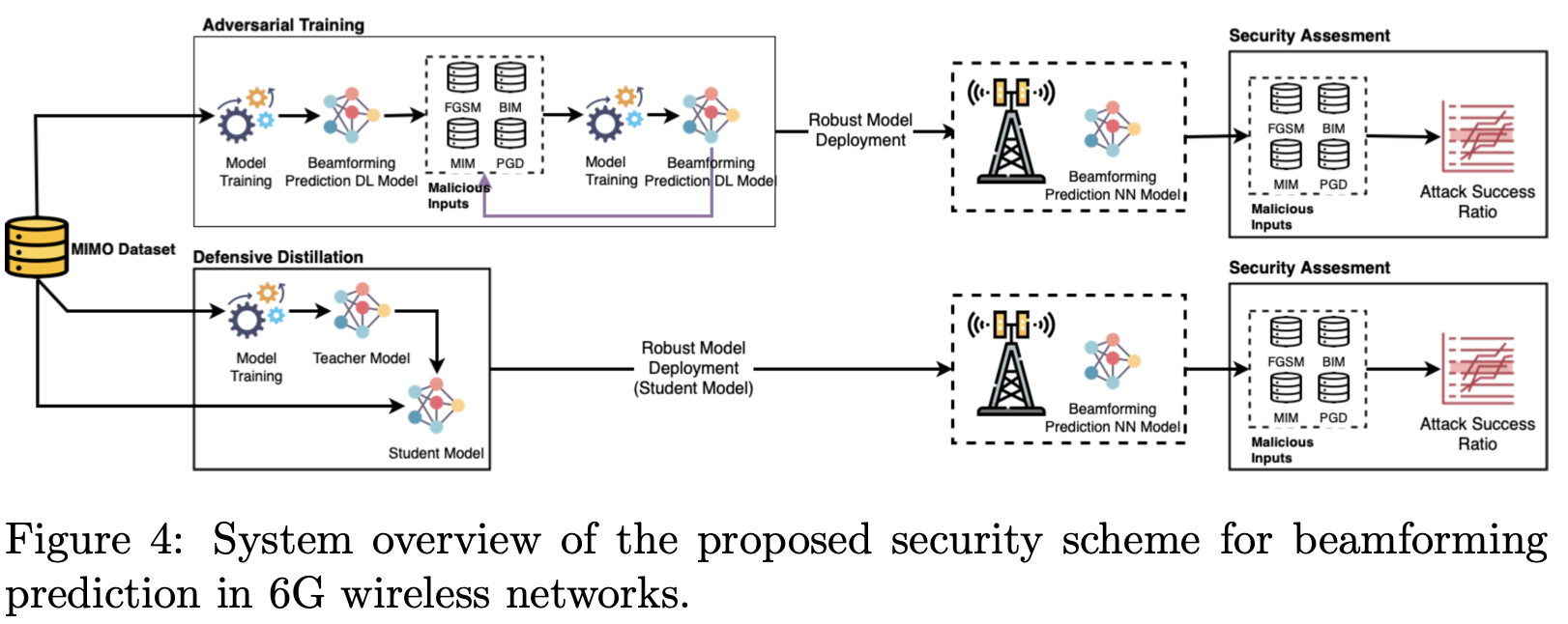
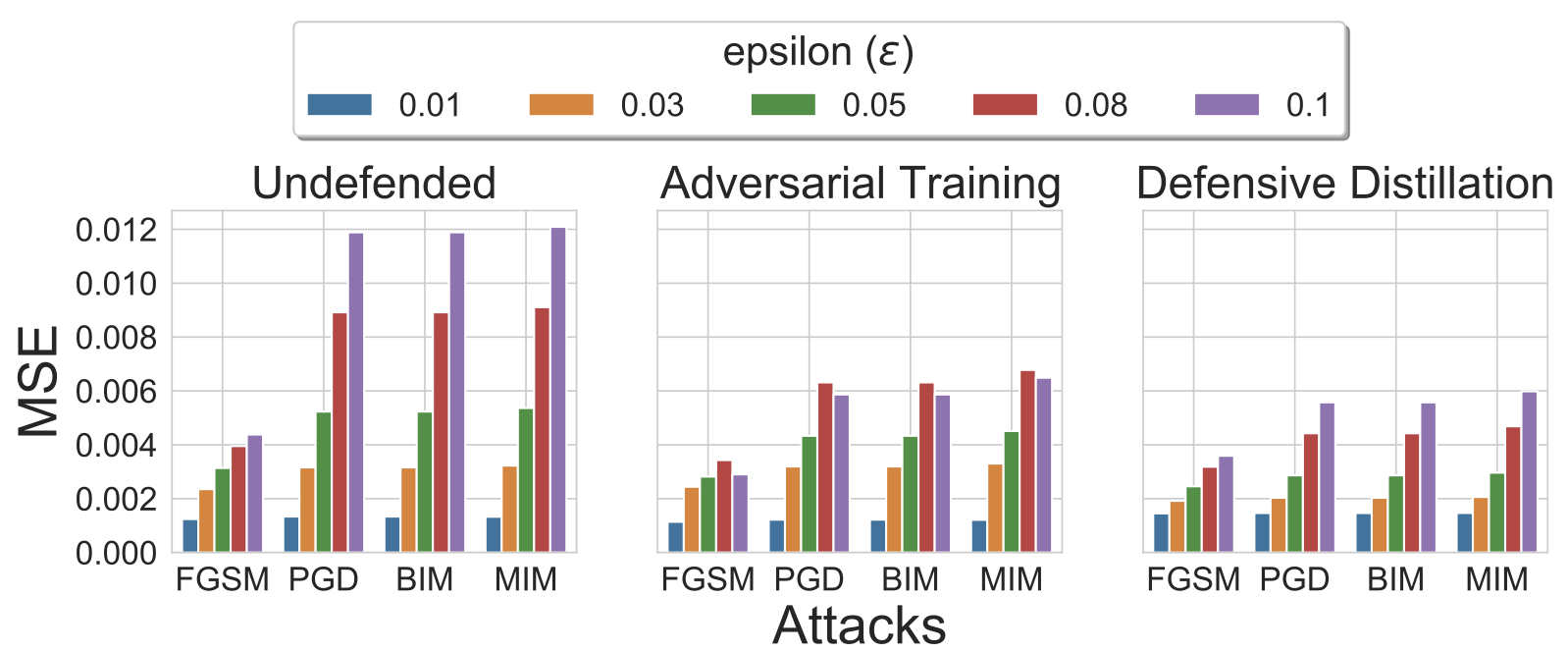
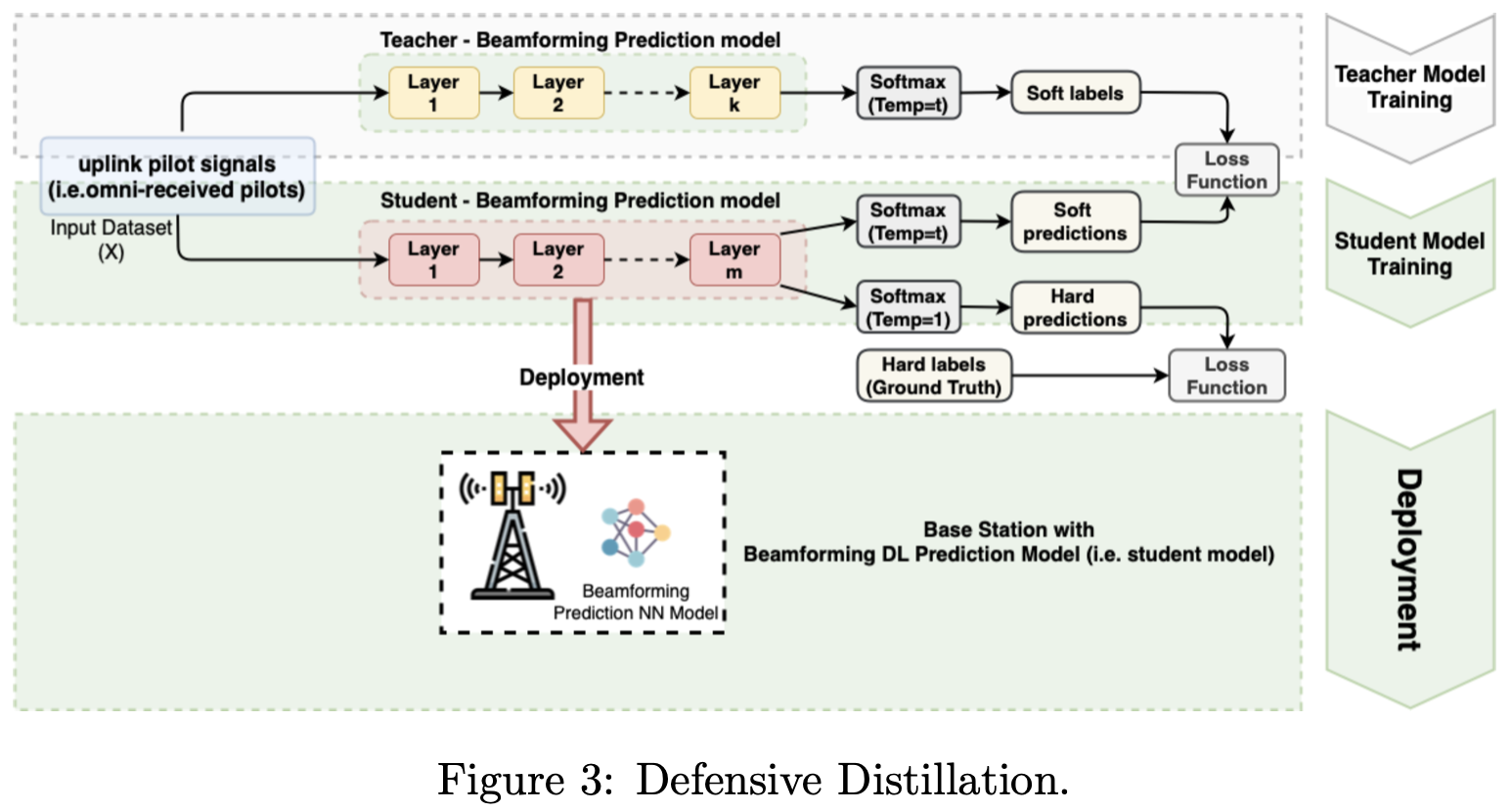